Nervous System: Audrey and the Dragon: The History of Voice Recognition Technology
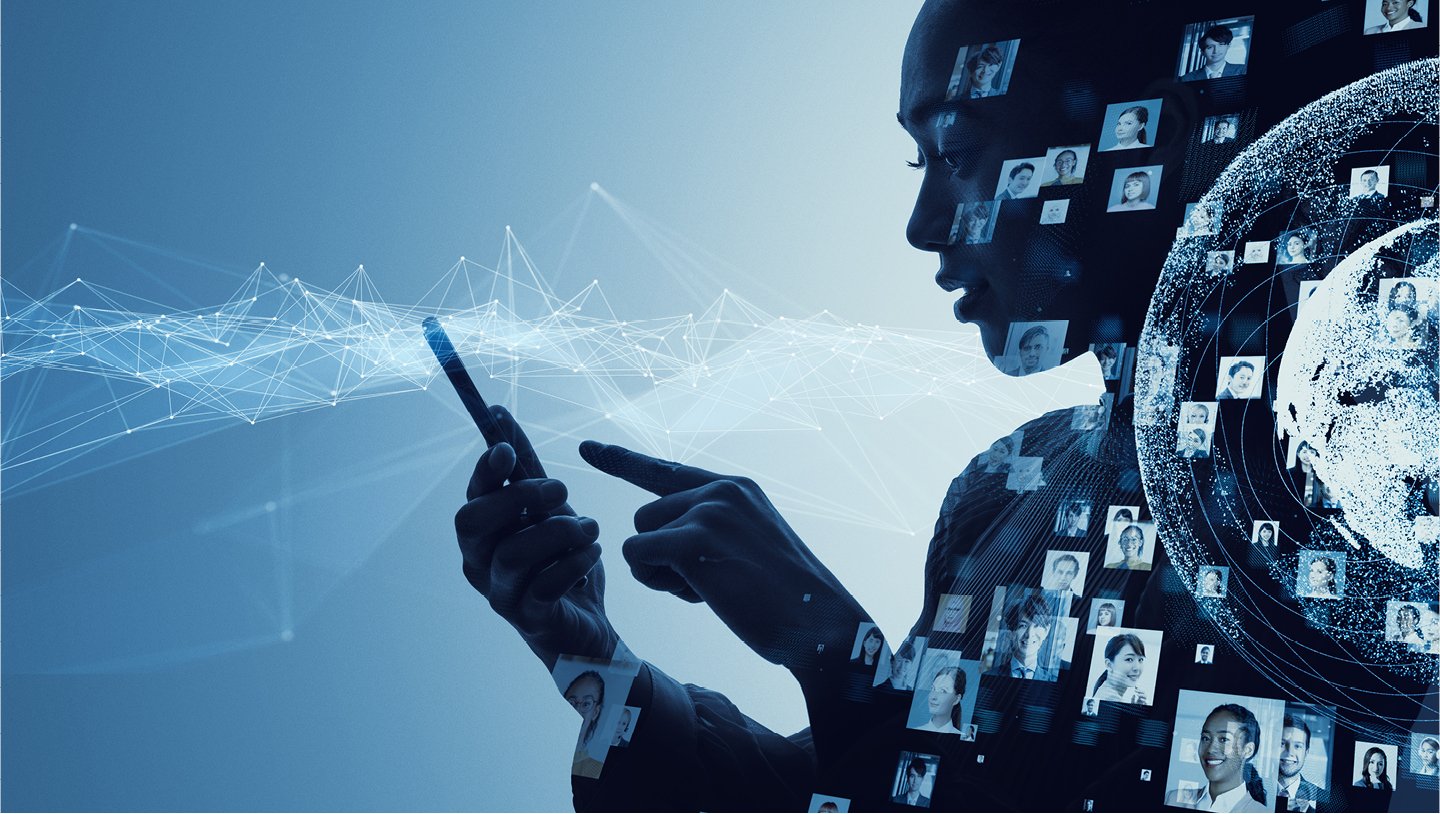
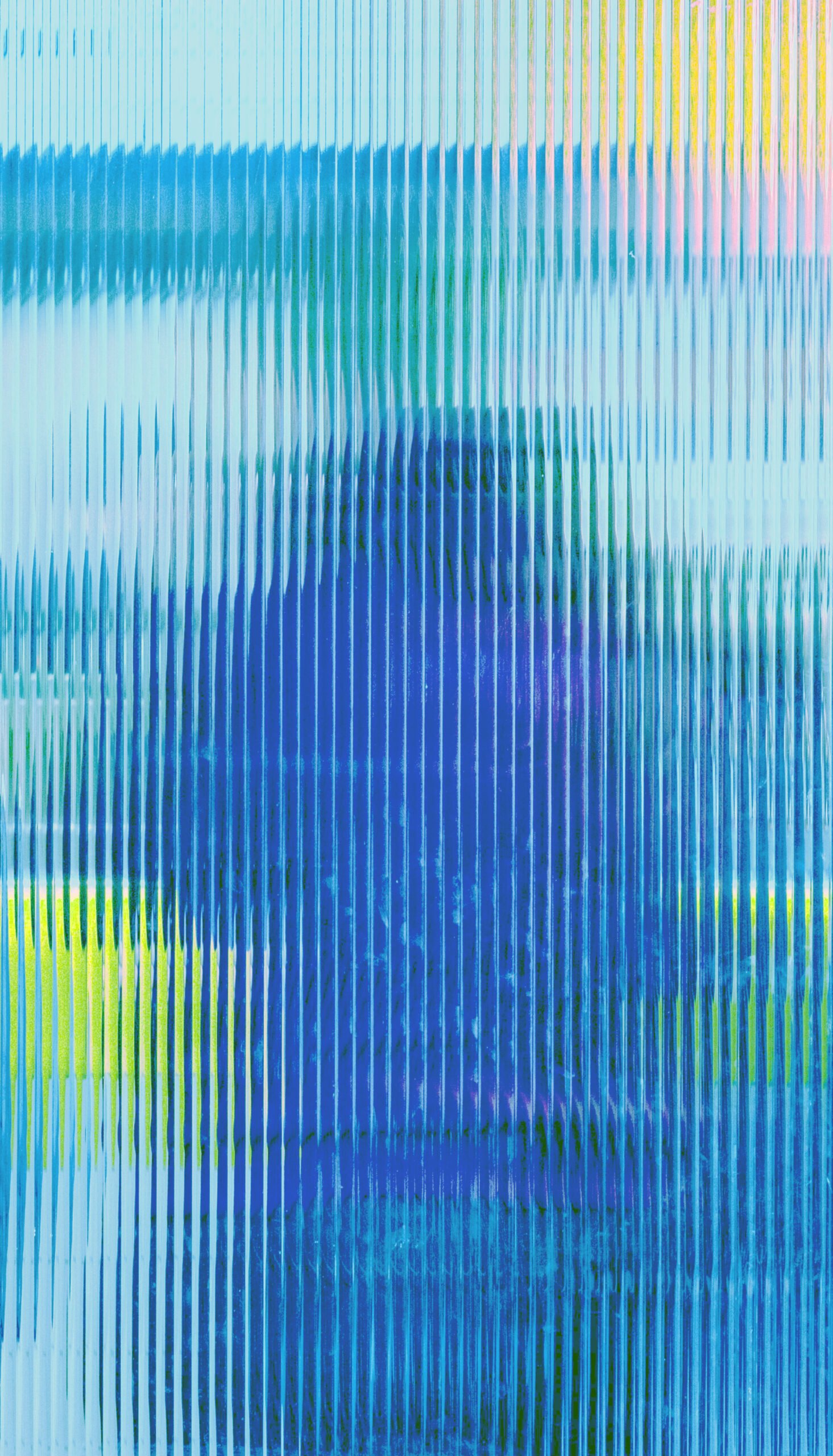
BRG is home to renowned thought leaders and experts considered authorities in their fields of work. Our timely research and perspectives provide analysis and insights on the most important issues facing the industries and organizations we serve.
The path leading to the likes of Siri and Alexa was long and winding. In this month’s history of cybersecurity, David Kalat looks back to the beginnings of voice recognition technology, from Bell’s Automatic Digit Recognition machine to projects from DARPA funding.
Modern voice recognition technology has become so ubiquitous that it is easy to forget the extraordinary engineering marvels it reflects. Although it is now routine to command smart devices using our voices, the path leading to the likes of Siri and Alexa was long and winding and crossed two major milestones in its early evolution.
The earliest voice recognition technologies were crude and limited by today’s standards, but they were critical in laying the groundwork for further advancements. By the 1970s, scientists began combining the early speech-recognition tools with clever statistical insights that brought about the first practical applications of the technology.
Scientists at Bell Laboratories invented the first known voice-recognition device in 1952. The Automatic Digit Recognition machine (or “Audrey”) was a breakthrough creation with just a single function: to recognize the sound of a human speaker saying any digit between zero and nine. Telephone companies were looking to automate functions performed by human operators, and a computer system capable of recognizing a caller’s spoken request for a given telephone number or extension theoretically could replace some or all of the human switchboard staffing.
There was nothing simple about Audrey. The massive contraption was larger than a person and consumed substantial power, and its working was analog, rather than a software algorithm.
Audrey’s accuracy varied with the speaker’s voice. Audrey favored its creator and could recognize his spoken digits with about 90 percent accuracy, but that precision fell off with other speakers. The more Audrey “worked” with a given speaker and adjusted to that speaker’s voice, the more accurate she became. Despite this capacity for learning, Audrey was not a functional replacement for human switchboard operators, who were faster, more precise, and ultimately cheaper than the machine.
Other inventions, developments, and improvements followed in Audrey’s wake, but the next major conceptual leap occurred under the auspices of the Department of Defense. In the 1970s, the Defense Advanced Research Projects Agency (DARPA) provided funding for the Speech Understanding Research Program. Crucially, engineers working on the DARPA initiative incorporated a fundamental, if counterintuitive, aspect of information science.
Back in 1951, Claude Shannon, the so-called “Father of Information Theory,” had conducted a famous demonstration with the help of his wife, Betty. Betty’s role was to guess, one letter at a time, the sequence of letters on a randomly selected page of a pulp detective novel. After the difficulty of correctly guessing the first several letters, Betty’s accuracy in predicting subsequent letters drastically improved, to the point that she could correctly predict entire sequences of letters—whole words and phrases. As Shannon illustrated, human language is largely redundant, and each successive word serves to close off the probability of which words could come next. Basic statistical information about grammar, vocabulary, word choice, and so on can be used to predict the content of any message from relatively small samples of that message.
Incorporating this concept into voice recognition systems meant equipping the software with statistical models of language, as well as advanced sensors for hearing and parsing the individual phonemes of speech. This was a controversial development, and many engineers working on voice recognition systems objected that the statistical modeling approach was misguided and that only advancements in artificial intelligence could improve speech recognition tools.
One of the most hyped of the DARPA speech recognition projects was “Harpy,” a system designed at Carnegie Mellon in 1972. Harpy could recognize over a thousand words, comparable to the vocabulary of a three-year-old child and a substantial improvement over other systems at the time, which could recognize fewer than twenty words. Harpy also could recognize complete sentences.
Carnegie Mellon’s software used language models to statistically evaluate the sounds it perceived within a linguistic framework of what made sense. One approach to mathematically modeling a linguistic framework involved the statistical concept of Hidden Markov models. These tools evaluate patterns of observed activity to draw conclusions about their unobservable causes and/or consequences. For example, an observer may not know the mental state of a friend but can observe her friend’s sequential actions on the understanding that actions are guided by mood. Hidden Markov models provide a relatively simple way to model this kind of sequential data.
As it happened, James K. Baker, a rising force in the world of speech recognition technology, was finishing his PhD thesis at Carnegie Mellon during the DARPA-funded research boom. In his landmark 1975 dissertation, “Stochastic Modeling as a Means of Automatic Speech Recognition,” Baker explored the uses of Hidden Markov models to recognize words from unrecognized sounds. This foundational research led to the first commercially viable speech recognition software.
In 1982, Baker and his wife, Janet MacIver Baker, formed Dragon Systems Inc. In 1997, Dragon released the first consumer-grade commercial voice recognition product, Dragon NaturallySpeaking. This software’s selling point was that, for the first time in decades of speech recognition research and development, the user did not need to speak haltingly with unnatural pauses for the benefit of the machine. Dragon’s software was the first to process continuous natural speech and remains in use today.
Find out more at Legaltech News.
The views and opinions expressed in this article are those of the author and do not necessarily reflect the opinions, position, or policy of Berkeley Research Group, LLC or its other employees and affiliates.
Related Professionals
Related Services
Related Industries
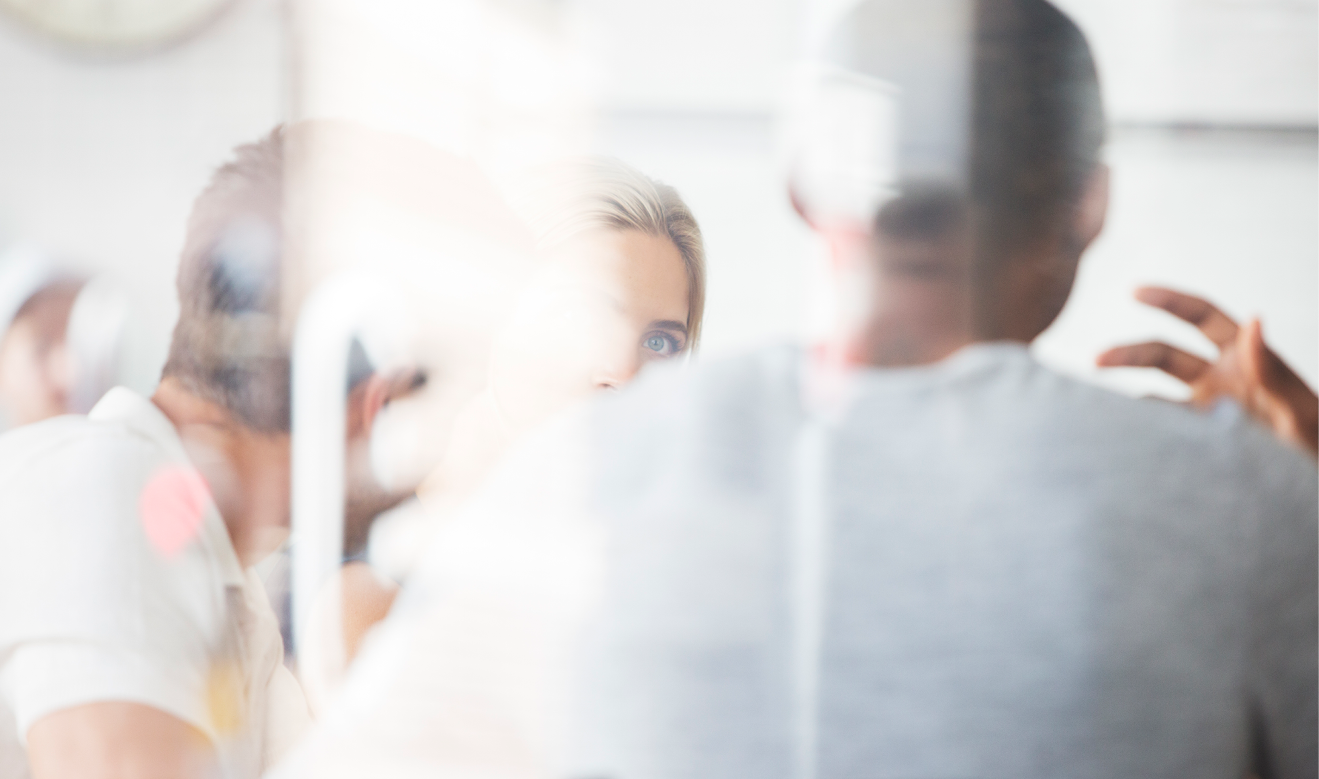
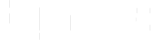
Prepare for what's next.
ThinkSet magazine, a BRG publication, provides nuanced, multifaceted thinking and expert guidance that help today’s business leaders adopt a more strategic, long-term mindset to prepare for what’s next.